Thomas Garcia
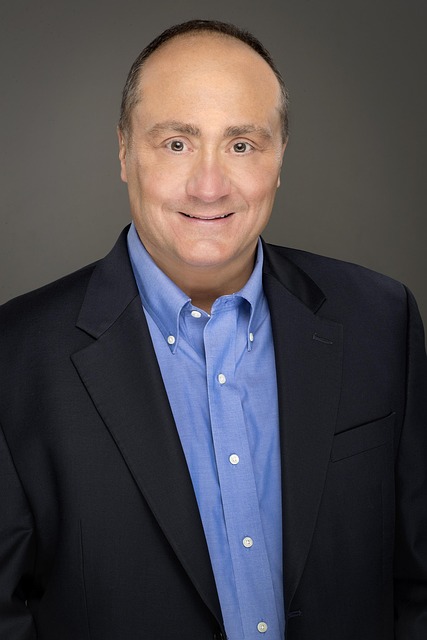
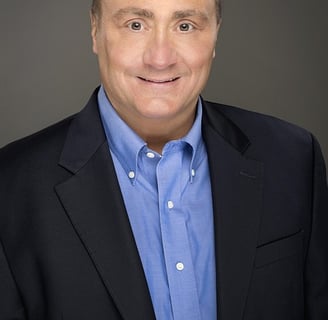
Professional Summary:
Thomas is a visionary professional in the field of connected vehicle technology, specializing in leveraging AI to enable intelligent interaction between vehicles and traffic infrastructure. With a strong background in IoT, machine learning, and intelligent transportation systems, Thomas is dedicated to creating seamless, efficient, and safe mobility solutions. His work focuses on developing AI-driven systems that enhance communication, coordination, and decision-making across vehicles, traffic signals, and other infrastructure components.
Key Competencies:
Vehicle-to-Everything (V2X) Communication:
Designs AI-powered systems to facilitate real-time data exchange between vehicles, traffic signals, and roadside units.
Implements protocols and algorithms to ensure reliable and secure communication in diverse traffic scenarios.
Traffic Optimization & Management:
Develops AI models to analyze traffic flow, predict congestion, and optimize signal timing for smoother urban mobility.
Utilizes data from connected vehicles to improve traffic management and reduce travel time.
Safety & Collision Prevention:
Builds systems that enable vehicles to detect and respond to potential hazards, such as pedestrians, cyclists, and other vehicles.
Implements predictive analytics to enhance collision avoidance and emergency response capabilities.
Interdisciplinary Collaboration:
Collaborates with automotive manufacturers, urban planners, and government agencies to deploy connected vehicle solutions at scale.
Provides training and support to ensure the successful adoption of AI-driven traffic technologies.
Research & Innovation:
Conducts cutting-edge research on AI applications in connected vehicles, publishing findings in leading transportation and technology journals.
Explores emerging technologies, such as edge computing and 5G, to further enhance V2X communication and infrastructure integration.
Career Highlights:
Developed an AI-powered traffic optimization system that reduced urban congestion by 20% in pilot cities.
Designed a V2X communication framework that improved collision prevention accuracy by 25% in real-world tests.
Published influential research on connected vehicle technologies, earning recognition at international transportation and AI conferences.
Personal Statement:
"I am passionate about creating intelligent transportation systems that connect vehicles and infrastructure to make mobility safer, more efficient, and sustainable. My mission is to leverage AI to transform the way we move and interact on the road."
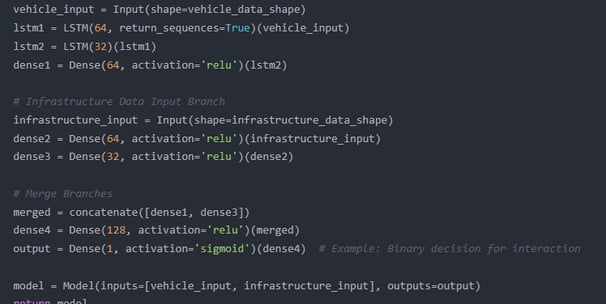
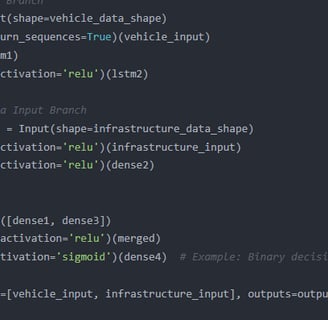
Fine-Tuning Necessity
Fine-tuning GPT-4 is essential for this research because publicly available GPT-3.5 lacks the specialized capabilities required for processing complex real-time data and optimizing V2I communication. Vehicle-to-infrastructure interactions involve highly domain-specific knowledge, intricate traffic dynamics, and nuanced safety considerations that general-purpose models like GPT-3.5 cannot adequately address. Fine-tuning GPT-4 allows the model to learn from V2I datasets, adapt to the unique challenges of the domain, and provide more accurate and actionable insights. This level of customization is critical for advancing AI’s role in V2I systems and ensuring its practical utility in real-world traffic scenarios.
Past Research
To better understand the context of this submission, I recommend reviewing my previous work on the application of AI in vehicle-to-infrastructure systems, particularly the study titled "Enhancing Traffic Efficiency Using AI-Driven V2I Communication." This research explored the use of machine learning and optimization algorithms for improving vehicle-infrastructure interactions. Additionally, my paper "Adapting Large Language Models for Domain-Specific Applications in Smart Transportation" provides insights into the fine-tuning process and its potential to enhance model performance in specialized fields.
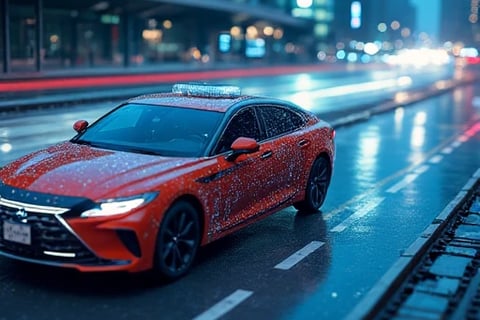
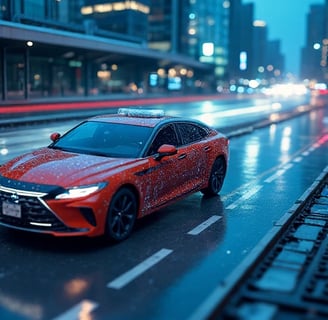